Machine learning is used in nearly all of our projects. Automating tedious and time-consuming tasks not only allows for the curation of large datasets with increased statistical power of analyses, but also performs tasks that are difficult for human (especially without extensive training). We build automated pipelines for processing multicell and whole brain recordings from C. elegans. The pipeline includes neuron detection, tracking, and identification, as well as signal denoising (e.g. for calcium transients). This work will allow us to look at the changes of brain dynamics under a variety of conditions. We also build pipelines to extract behavior of the worms from massive datasets, with tasks including individual worm tracking, identification, and extractions of behavior dynamics.
Highlighted Lab Publications
[1] Chaudhary, S., Lee, S.A., Li, Y., Patel, D., Lu, H., Graphical-model framework for automated annotation of cell identities in dense cellular images. eLife. 2021. https://doi.org/10.7554/eLife.60321
[2] Chaudhary, S., Moon, S. & Lu, H., Fast, efficient, and accurate neuro-imaging denoising via supervised deep learning. Nat Commun. 2022, 13. https://doi.org/10.1038/s41467-022-32886-w
[3] Bates K, Le KN, Lu H., Deep learning for robust and flexible tracking in behavioral studies for C. elegans. PLOS Computational Biology. 2022, 18. https://doi.org/10.1371/journal.pcbi.1009942
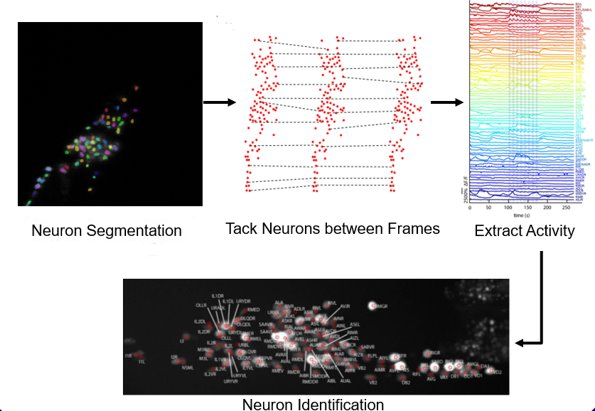